The Growing Acceptance of CAE
Simulation is being used for lawsuits in court, treating schizophrenia, use in middle school education and in deep learning.
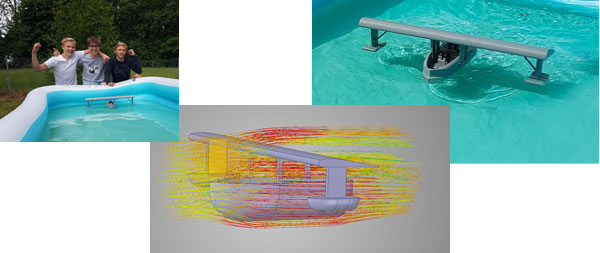
Students used ANSYS Discovery Live and high-performance computing to help simulate their flying boat design. Image courtesy of UberCloud.
Latest in High–performance Computing HPC
High–performance Computing HPC Resources


Simr
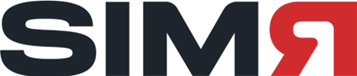
Latest News
December 18, 2018
With the following four UberCloud case studies we are demonstrating the growing acceptance of computer-aided engineering (CAE), especially in new user communities and application areas. Major reasons for this trend are continuous improvements in user-friendly CAE software, the ongoing price/performance improvement in high-performance computing (HPC), and easy and affordable access to powerful computing in the cloud.
We demonstrate how 1) a US law firm applied multi-physics building simulations using HPC to prove building defects at a client’s property; 2) how a class of ninth-graders in Norway designed and built a flying boat with novel CAE software; 3) how the Indian National Institute of Mental Health was able to replace a highly risky brain-invasive operation for Schizophrenia treatment with a revolutionary non-invasive, low-risk treatment based on HPC; and 4) how deep learning for fluid flow prediction in the cloud can potentially reduce CFD simulations from minutes to milliseconds. All four projects have been performed by UberCloud, and sponsored by Hewlett Packard Enterprise and Intel. The first two projects received international awards during the 2018 Supercomputing Conference in Dallas.
Simulating Moisture Transfer in a Residential Condo Tower
“This study demonstrates that, in general, multi-physics building simulation using HPC Cloud computing is accessible, affordable, and beneficial for our clients.”
One year ago, PBBL Law Offices in Las Vegas/Orlando approached UberCloud and Fraunhofer Institute for Building Physics asking for HPC support in a lawsuit dealing with a twin tower residential condominium. An extensive expert investigation established exterior plaster failure, water intrusion at improper window and roof installations, and high interior humidity levels with apparent biological growth (ABG) observed on interior walls, baseboards and between layers of interior gypsum board at unit partitions. A mechanical engineering evaluation found that the air conditioning units (serving each condominium) were improperly sized to adequately manage humidity. PBBL Law, for the first time, chose HPC modeling to determine whether damage was caused by moisture transfer through the plaster-coated exterior walls or negative pressure in the living units.
Parametric Study
The project team used WUFI Plus, a hygrothermal building simulation software from Fraunhofer Institute for Building Physics (IBP), based on coupled heat and moisture transport across building components. With different compute instances in Microsoft Azure, a benchmark checked the computing times to identify the best system configuration. Tested were the 2-core DS11, 4-core DS12, 8-core DS13, and 16-core DS14 instances for best price/performance. The simulation period for the building model was one year. Table 1 shows the elapsed computation time on the different machines.
Conclusions
For this specific project, the coating damages are not related to high indoor air relative humidity but to vapor permeability of the coating and the driving rain leakage behind coating. Indoor climate humidity and mold issues are not related to coating permeability but to HVAC (heating, ventilation and air conditioning) equipment and infiltration of unconditioned and untreated outside air.
For the final parameter study, 16-core DS14 was chosen, 8 simulations at a time, with a total of 583 simulations. Because parameter studies consist of independent simulations, we were able to run 16 simulations in parallel on two DS14 and 32 simulations on four DS14, reducing the total simulation time from about 43 days on the user’s workstation to less than two days in the Microsoft Azure Cloud, a whopping speed-up of 20+!
HPC cloud computing also enables the prediction of future performance of buildings by simulation for a broad range of input parameters in a reasonable time period, allowing invasive/destructive building forensics to be reduced. Together with the ability to separate design, material and workmanship deficiencies, the design process, potential forensic investigations or litigation can draw huge benefits from using HPC cloud computing with hygrothermal building simulation. Readers can download the detailed UberCloud Case Study #207, which recently won the 2018 HPCwire Editors’ Choice Award for Best Use of HPC in the Cloud and the 2018 Hyperion Innovation Excellence from Hyperion and the HPC User Forum.
CAE in Personalized Noninvasive Clinical Treatment of Neurological Disorders in the Brain
UberCloud use case #200 demonstrates how India’s National Institute of Mental Health and Neuro Sciences (NIMHANS) simulated neuromodulation to treat Schizophrenia — and potentially Parkinson’s disease, depression, and other brain disorders. NIMHANS was able to replace the current highly risky procedure of brain-invasive operations with an innovative, potentially ambulant technique of non-invasive low-risk treatment based on HPC that is more affordable. With the addition of HPC, clinicians can now precisely and non-invasively target regions of the brain without affecting major parts of the healthy brain! The HPC simulations have been collaboratively performed by NIMHANS, Dassault Systemes SIMULIA, and the Advania Data Centers HPC cloud.
The Problem
Neuromodulation refers to neural activity via an artificial stimulus such as an electrical current or a chemical agent. It may involve (highly risky) invasive approaches such as spinal cord or deep brain stimulation where electrodes are implanted directly on the nerves to be stimulated. It may also be performed non-invasively using methods such as electrical stimulation in which external electrodes induce the required neural activity changes without the need for surgical implantation, but in which low intensity (mA) electrical currents are applied to the head via scalp-mounted electrodes, as shown in Figure 2. This method may be used to increase cortical brain activity in specific brain areas that are under aroused, or alternatively decrease activity in areas that are overexcited. This procedure is simple, affordable, portable and can be applied in an ambulant treatment, and the human is fully conscious and experiences minimal discomfort.
HPC Brain Simulation in the Advania Data Centers Cloud
Multi-physics technology allowed us to simulate deep brain stimulation by placing two sets of electrodes on the scalp to generate temporal interference deep inside the gray matter of the brain. Customization in post processing made this methodology available to the clinician in real time and reduced overall computational effort. Doctors can choose two pre-computed electrical fields of an electrode pair to generate temporal interference at specific regions of the gray matter of the brain.
A high-fidelity finite element human head model was considered including skin, skull, cerebrospinal fluid (CSF), sinuses, as well as gray and white matter, which demanded high-performance computing resources to try various electrode configurations. Access to the HPE cluster at Advania and SIMULIA’s Abaqus 2017 code in an UberCloud HPC container empowered us to simulate numerous configurations of electrode placements and sizes. This also allowed us to study the sensitivity of electrode placements and sizes, which was not possible before on the end-user’s inhouse systems.
We ran 26 Abaqus jobs — each representing a different electrode configuration — on the Advania HPC cloud. Each job contained 1.8 million finite elements. On our own 16-core cluster, a single run took 75 minutes, whereas in the cloud a single run took 28 minutes on 24 cores. However, the real advantage comes from performing the 26 different electrical simulations all in parallel, reducing simulation time for all 26 simulations from 33 hours to 28 minutes, a speed-up factor of 70, just by using cloud. With this achievement, the patient can just wait for the results, the doctor identifies the best electrode configuration and fine tunes the patient’s remote control, all in an ambulant treatment that will be available soon for the masses.
This application demonstrates a breakthrough for deep brain stimulation in a non-invasive way, which has the potential to replace more painful/high-risk brain surgeries such as in Schizophrenia and Parkinson. The huge benefits of these HPC simulations are that:
- they predict the electrical current distribution with high resolution;
- they allow for personalized and quantifiable treatment;
- they facilitate electrode montage variations; and
- clinicians can devise the most effective treatment for a specific patient.
- Instead of a painful, high-risk and expensive operation this novel approach allows for an ambulant treatment.
You can download UberCloud Case Study #200 which received the 2018 Hyperion Innovation Excellence from Hyperion and the HPC User Forum.
Ninth Graders Design Flying Boats with ANSYS Discovery Live
This UberCloud use case #208 is about 14-year-old students from Torsdad Middle School in Sandvika, Norway, designing flying boats in ANSYS Discovery Live on Microsoft Azure’s NV6 GPU instances. The students 3D printed the boats and crowned their three-month tech course with a final competition for the best flying boat. Help was given by their physics teacher Ole Nordhaug, engineer Håkon Bull Hove from ANSYS channel partner EDRMedeso, and HPC Cloud service provider UberCloud.
The students used ANSYS Discovery Live, released in the first quarter of 2018, which provides real-time 3D simulation, tightly coupled with direct geometry modeling, to enable interactive design exploration and rapid product innovation. It is an interactive, multi-physics simulation environment in which users can manipulate geometry, material types and physics inputs, and see results instantly.
Hired by Fictional Company “FlyBoat”
In their tech course, the students got a taste of the work of an engineer. They were told that a fictional company called “FlyBoat” was planning to develop a combination of a boat and a sea plane. FlyBoat had hired them to do a concept study. Within some given parameters, like height, width and length, the students were free to innovate. Eventually, FlyBoat would pick one of the concepts for their design. To win the competition, the students had to come up with the best overall design, which required attention to many different aspects of boat and plane design. At the end of the semester, all the boats were 3D printed, and the students held sales presentations to convince a panel that their concept was indeed the best.
3D Modeling in Discovery Live
The students designed a 3D model of the boat in ANSYS Discovery Live. None of the students had prior experience with CAD modelling nor simulations, but they took the challenge head-on.
“The students learned the software impressively quickly. It was really inspiring to see what concepts they came up with”, says Håkon Bull Hove, an engineer at EDRMedeso. Together with teacher Ole Nordhaug, he demonstrated Discovery Live to the students and helped them with their simulations.
Perhaps the most challenging task of the project was to prove that the flying boats could indeed fly. To do this, the students performed aerodynamic analyses of the wing in ANSYS Discovery Live, examining both lift and drag. Their simulations not only proved that the wings had enough lift, but provided excellent visualization of the physics as well.
“It is much easier to understand foil theory when you see it live on your screen,” says teacher Ole Nordhaug. “To see such a spirit among the students was the most inspiring moment in the entire project.”
Using UberCloud Computing
During the whole course of the design and simulation project — from March to June — the students were supported by UberCloud, which each week provided 10 ANSYS Discovery Live environments sitting on 10 Azure 6-core Windows compute nodes, each equipped with 56GB and an NVIDIA Tesla M60 GPU for accelerating compute and NICE DCV real-time remote visualization. Cloud resources were located in Microsoft’s Azure datacenter in Amsterdam, which the students accessed instantly, with login and password, through their web browser.
This middle school project with 14-year-old students and their physics teacher in Norway is just another impressive demonstration of the current trend toward more user-friendly application software, combined with extremely fast HPC Cloud infrastructure (equipped with GPUs) available for everyone at their fingertips. ANSYS Discovery Live on the cloud isa big step forward toward “democratizing” high-performance computing and engineering simulation. Interested readers can download the case study #208 here.
Deep Learning for Fluid Flow Prediction in the Cloud
This UberCloud project #211 has been collaboratively performed by Jannik Zuern, Ph.D. student at University of Freiburg in Germany supported by Renumics GmbH for Automated CAE, and cloud resource provider Advania Data Centers. OpenFOAM and Renumics AI tools have been packaged into an UberCloud HPC software container.
Solving fluid flow problems using computational fluid dynamics (CFD) is demanding both in terms of computing power and simulation time. Artificial neural networks (ANN) learn complex dependencies between high-dimensional variables. This ability is exploited in a data-driven approach to CFD that is presented in this case study. An ANN is applied to predicting the fluid flow given only the shape of the object. The goal of the approach is to apply an ANN to solve fluid flow problems to significantly decrease time-to-solution while preserving much of the accuracy of a traditional CFD solver. Creating a large number of simulation samples is paramount to let the neural network learn dependencies between simulated design and the flow field around it.
This project was established to explore the benefits of additional cloud computing resources on Advania Data Centers that can be used to create a large amount of simulation samples in parallel in a fraction of the time a desktop computer would need to create them. We wanted to explore whether the overall accuracy of the neural network can be improved with more samples being created in the UberCloud HPC/AI container based on Docker Community Edition and OpenFOAM CFD software — and then used during the training of the neural network.
Automated CFD Workflow Overview
To create the simulation samples automatically, a comprehensive four-step deep learning workflow was established, as shown in Figure 6.
- Step 1: Random 2D shapes are created, which have to be diverse enough to let the neural network learn the dependencies between different kinds of shapes and their respective surrounding flow fields.
- Step 2:The shapes are meshed and added to an OpenFOAM simulation template.
- Step 3: The simulation results are post-processed using the open-source visualization tool ParaView. The flow-fields are resampled on a rectangular grid to simplify the information processing by the neural net.
- Step 4:Both the simulated design and the flow fields are fed into the input of the neural network.
After training, the neural network is able to infer a flow field merely from seeing the to-be-simulated design.
Training Results
As a first step, we compared the time it takes to create the samples on the desktop workstation with the time it takes to create the same number of samples on UberCloud/Advania. On the desktop it took 13h 10min to create the 10,000 samples. In the UberCloud/Advania it took 2h 4min to create 10,000 samples, a speedup of 6.37x.
A total of 70,000 samples were created. We compared the losses and accuracies of the neural network for different training set sizes. To determine the loss and the accuracy of the neural network, we first defined “loss of the neural network prediction,” which describes the difference between the prediction of the neural network and the fully simulated results. Similarly, the level of accuracy that the neural network achieves had to be described. For details about the ‘loss’ and the ‘level of accuracy’ see the complete case study.
The more different samples the neural network processes during the training, the better and faster it is able to infer a flow velocity field from the shape of the simulated object suspended in the fluid. Figure 8 illustrates the difference between the flow field (left) and the predicted flow (right) for one exemplary simulation after 300,000 training steps, showing no difference between the flow fields.
We were able to demonstrate a mantra among machine learning engineers: The more data the better. We showed that the training of the neural network is substantially faster using a large dataset of samples compared to smaller datasets. The metrics for measuring the accuracies of the neural network predictions exhibited higher values for larger numbers of samples. The overhead of creating high volumes of additional samples can be effectively compensated by high-performance containerized computing node. A speed-up of more than 6x compared to a state-of-the-art desktop workstation allows creating the tens of thousands of samples needed for the neural network training process in a matter of hours instead of days. To train more complex models (e.g. for 3D flow models) much more training data will be required. Thus, software platforms for training data generation and management as well as flexible compute infrastructure will become increasingly important.
More Simr Coverage
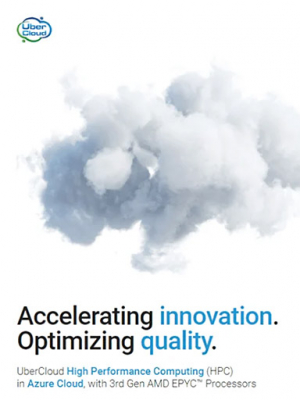
For More Info
Wolfgang Gentzsch is co-founder and president of UberCloud, the community, marketplace, and software provider for engineers and scientists to discover, try, and buy complete hardware/software solutions in the cloud. Wolfgang is a passionate engineer and high-performance engineering computing veteran with 40 years of experience as a researcher, university professor, and serial entrepreneur. He can be reached at wolfgang.gentzsch@theubercloud.com or on LinkedIn. Extended case studies of these examples can be downloaded from the UberCloud site.
Subscribe to our FREE magazine,
FREE email newsletters or both!Latest News
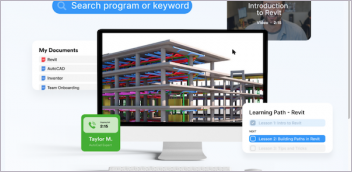
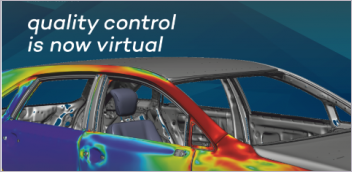
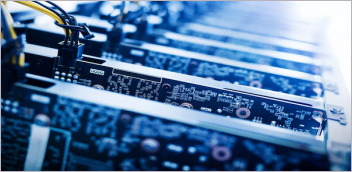
